Introduction
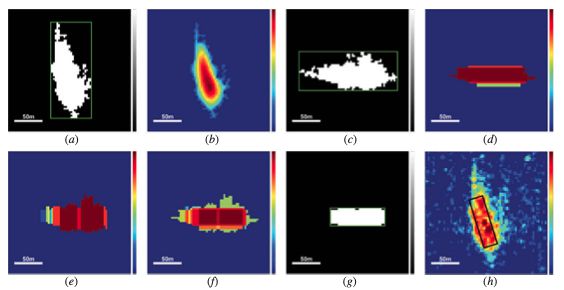
Data
The data used in this project is a Sentinel-1 SAR VV-polarized single band image from the 29th of March 2021, downloaded from Google Earth Engine.
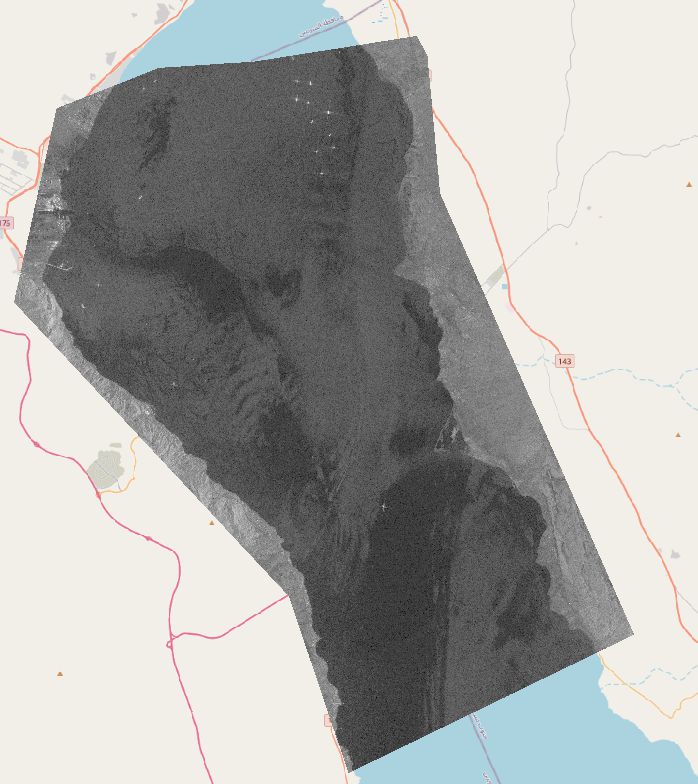
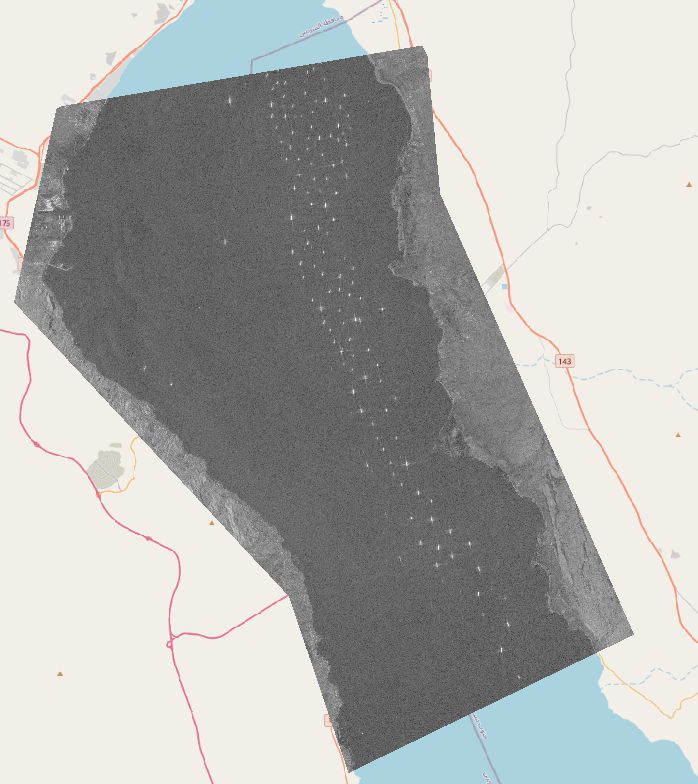
Preprocessing for Morphology
First, a small subset of several ships es extracted, since a small image is easier to work with during the testing phase.
In order to prepare the data for the binary mathematical morphology, the values of the image are reclassified based on a threshold, which is manually chosen. The threshold chosen is 0, being the divider between negative values (bo reflectance to sensor) and positive values (where a return is measured). The resulting image still has some error pixels and the ships are quite deformed.
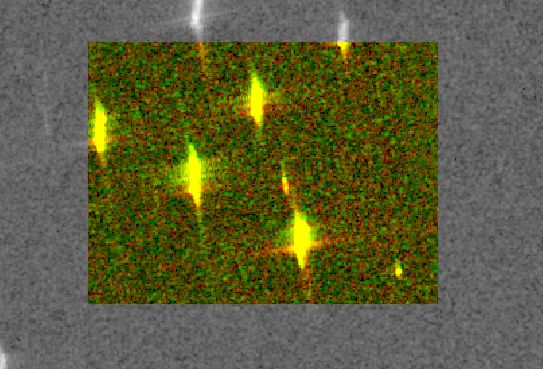
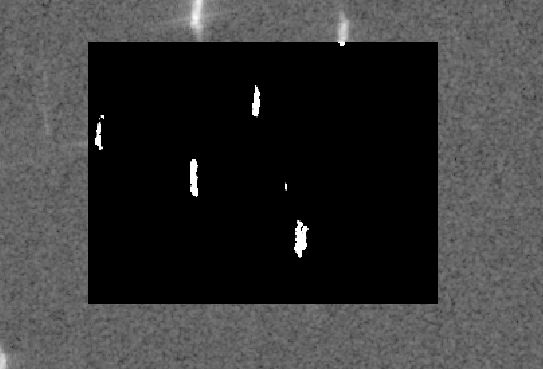
Workflow
As outlined in the paper, the workflow starts with a multi-angular erosion, followed by a thresholding for their specific purpose. Following a rotation, multi-scale openings and closings are performed, which are then added. The final result, after another thresholding, is a minimum bounding box of the ship.
The workflow relies heavily on a thresholding via CFAR, after some testing it becomes clear that the implementation unfortunately exceeds the scope of this exercise. Since the paper mentions some alternatives to CFAR, namely Otsu’s thresholding method, this was implemented instead.
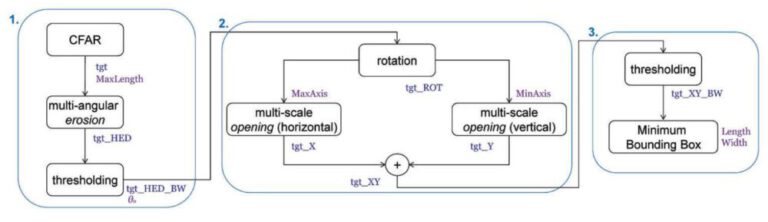
Notebook
This Jupyter Notebook recreates the workflow of the paper on a subset test image than the original Suez Canal image. As outlined in the notebook, compromises needed to be made with regards to certain steps since they were not reproducible. Additionally, some thresholds that were calculated adaptively in the paper were selected manually. At the end, a ship mask is still obtained.
The data and all notebooks, including the notebook on the full dataset, can be found on GitHub.
Implementing Workflow¶
Paper: https://www.tandfonline.com/doi/full/10.1080/2150704X.2016.1226522
Workflow:
T.o.C.:
- Preparation 0.1 Loading Data 0.2 Defining thresholding and plot functions
- Step 1: 1.1 Thresholding 1.2 Multi-Angular erosion 1.3 Thresholding
- Step 2: 2.1 Rotation 2.2 Multi-Scale Opening 2.3 Addition of results
- Step 3: 3.1 Thresolding 3.2 Minimim Bounding Boxes
- Export Results 4.1 Create Ship mask 4.2 Write Ship Mask to Band
import scipy.ndimage
import rasterio
import skimage.morphology
from matplotlib import pyplot as plt
import matplotlib
import numpy as np
import random
import matplotlib.pyplot as plt
from skimage import data
from skimage.filters import threshold_otsu
from numpy.ma import masked_array
import numpy.ma
from skimage.morphology import (erosion, dilation, opening, closing, white_tophat)
from skimage.morphology import black_tophat, skeletonize, convex_hull_image # noqa
from skimage.morphology import disk
import numpy as np
import numpy.ma as ma
input_file_path = "data/test_data_crop.tif"
# Read image and raster
src = rasterio.open(input_file_path)
array = src.read(1)
plt.imshow(array,cmap="gray")
<matplotlib.image.AxesImage at 0x7efdea067490>
0.2 Defining thresholding and plot functions¶
def plot_comparison(original, filtered, filter_name):
fig, (ax1, ax2) = plt.subplots(ncols=2, figsize=(15, 10), sharex=True,
sharey=True)
ax1.imshow(original, cmap=plt.cm.gray)
ax1.set_title('original')
ax1.axis('off')
ax2.imshow(filtered, cmap=plt.cm.gray)
ax2.set_title(filter_name)
ax2.axis('off')
def otsu(array,plot):
image = array
thresh = threshold_otsu(image)
binary = image > thresh
if plot:
image_masked = np.multiply(image,binary)
fig, axes = plt.subplots(ncols=3, figsize=(15, 4))
ax = axes.ravel()
ax[0] = plt.subplot(1, 3, 1)
ax[1] = plt.subplot(1, 3, 2)
ax[2] = plt.subplot(1, 3, 3, sharex=ax[0], sharey=ax[0])
ax[0].imshow(image, cmap=plt.cm.gray)
ax[0].set_title('Original')
ax[0].axis('off')
ax[1].hist(image.ravel(), bins=256)
ax[1].set_title('Histogram')
ax[1].axvline(thresh, color='r')
ax[2].imshow(binary, cmap=plt.cm.gray)
ax[2].set_title('Thresholded Mask')
ax[2].axis('off')
plt.show()
#return image values only for True pixels
return(binary)
# test functions
plot_comparison(array, np.multiply(array,otsu(array,True)), "thresholded")
mask_step_1 = otsu(array,False)
image_masked_step_1 = np.multiply(array,mask_step_1)
1.2 Multi-Angular Erosion¶
Instead of 5° steps, 4 steps of 45° are implemented. (Note: how to get 5° slope in np?)
plot_comparison(array,erosion(image_masked_step_1,skimage.morphology.rectangle(10,5)),"Erosion Rectangle")
se_1 = np.array([[0,0,0,0,0,1,0,0,0,0],
[0,0,0,0,0,1,0,0,0,0],
[0,0,0,0,0,1,0,0,0,0],
[0,0,0,0,0,1,0,0,0,0],
[0,0,0,0,0,1,0,0,0,0],
[0,0,0,0,0,1,0,0,0,0],
[0,0,0,0,0,1,0,0,0,0],
[0,0,0,0,0,1,0,0,0,0],
[0,0,0,0,0,1,0,0,0,0],
[0,0,0,0,0,1,0,0,0,0],
])
se_2 = np.array([[1,0,0,0,0,0,0,0,0,0],
[0,1,0,0,0,0,0,0,0,0],
[0,0,1,0,0,0,0,0,0,0],
[0,0,0,1,0,0,0,0,0,0],
[0,0,0,0,1,0,0,0,0,0],
[0,0,0,0,0,1,0,0,0,0],
[0,0,0,0,0,0,1,0,0,0],
[0,0,0,0,0,0,0,1,0,0],
[0,0,0,0,0,0,0,0,1,0],
[0,0,0,0,0,0,0,0,0,1],
])
se_3 = np.array([[0,0,0,0,0,0,0,0,0,0],
[0,0,0,0,0,0,0,0,0,0],
[0,0,0,0,0,0,0,0,0,0],
[0,0,0,0,0,0,0,0,0,0],
[0,0,0,0,0,0,0,0,0,0],
[1,1,1,1,1,1,1,1,1,1],
[0,0,0,0,0,0,0,0,0,0],
[0,0,0,0,0,0,0,0,0,0],
[0,0,0,0,0,0,0,0,0,0],
[0,0,0,0,0,0,0,0,0,0],
])
se_4 = np.array([[0,0,0,0,0,0,0,0,0,1],
[0,0,0,0,0,0,0,0,1,0],
[0,0,0,0,0,0,0,1,0,0],
[0,0,0,0,0,0,1,0,0,0],
[0,0,0,0,0,1,0,0,0,0],
[0,0,0,0,1,0,0,0,0,0],
[0,0,0,1,0,0,0,0,0,0],
[0,0,1,0,0,0,0,0,0,0],
[0,1,0,0,0,0,0,0,0,0],
[1,0,0,0,0,0,0,0,0,0],
])
se_multiangle = [se_1,se_2,se_3,se_4]
for se,degree in zip(se_multiangle,["90°","45°","0°","135°"]):
plot_comparison(image_masked_step_1,erosion(image_masked_step_1,se),"10px flat Erosion at "+degree)
1.3 Thresholding¶
Since the Multi-Angular step does influence the resuts at the end, a thresholding at this level is not strictly necessary. Still, they are visualized. Also, the thresold method differs from the one used in the paper, which is most likely the reason for the less than ideal result after thresholding
for se,degree in zip(se_multiangle,["90°","45°","0°","135°"]):
plot_comparison(image_masked_step_1,np.multiply(erosion(image_masked_step_1,se),erosion(otsu(array,False),se)),"Thresholded - 10px flat Erosion at "+degree)
2.1 Rotation¶
Here, the authors rotate the matrix so that the main orientation of clusters is vertical. Since the ships in this image are already mainly vertical, there is no need to rotate it.
2.2 Multi-Scale (Horizontal and Vertical) Opening¶
Since the scale is "hard-coded" into this recreation of the workflow, the same scale as in the first step is used.
# Horizontal Opening
# erosion
pass_1 = erosion(image_masked_step_1,se_3)
# closing
horizontal_opening = dilation(pass_1,se_3)
# Result
plot_comparison(image_masked_step_1,horizontal_opening,"Horizontal Opening")
# Vertical Opening
# erosion
pass_1 = erosion(image_masked_step_1,se_1)
# closing
vertical_opening = dilation(pass_1,se_1)
# Result
plot_comparison(image_masked_step_1,vertical_opening,"Vertical Opening")
2.3 Addition of results¶
addition = np.add(horizontal_opening,vertical_opening)
plot_comparison(image_masked_step_1,addition,"Addition of horizontal and vertical Opening")
addition_thresholded = np.multiply(addition,otsu(addition,False))
plot_comparison(addition,addition_thresholded,"Threshold of Addition")
3.1 Remove last pixels?!¶
final = dilation(addition_thresholded,skimage.morphology.square(2))
plot_comparison(addition_thresholded,final,"Another Erosion")
3.2 Minimum Bounding Box¶
ship_mask = ma.getmask(ma.masked_where(addition_thresholded < 0, addition_thresholded))
ship_mask = ship_mask.astype(int)
plt.imshow(ship_mask,cmap="gray")
<matplotlib.image.AxesImage at 0x7f5d9a9dbe90>
4.2 Write Ship Mask to Band¶
ToDo
Conclusion
4.1 Final Result – Original Workflow
The result obtain by following the workflow of the paper is not ideal, mostly because of the thresholding method. Therefore, another end result is created without the thresholding and some other optimized characteristics.
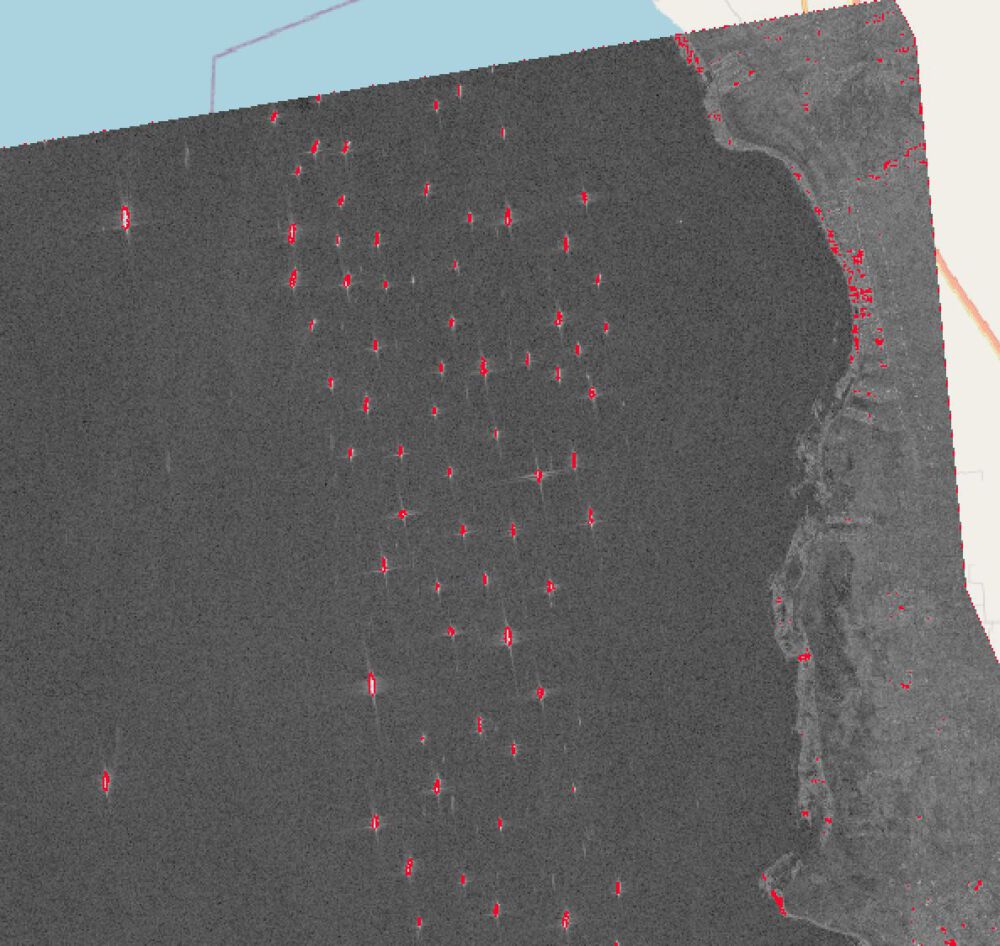
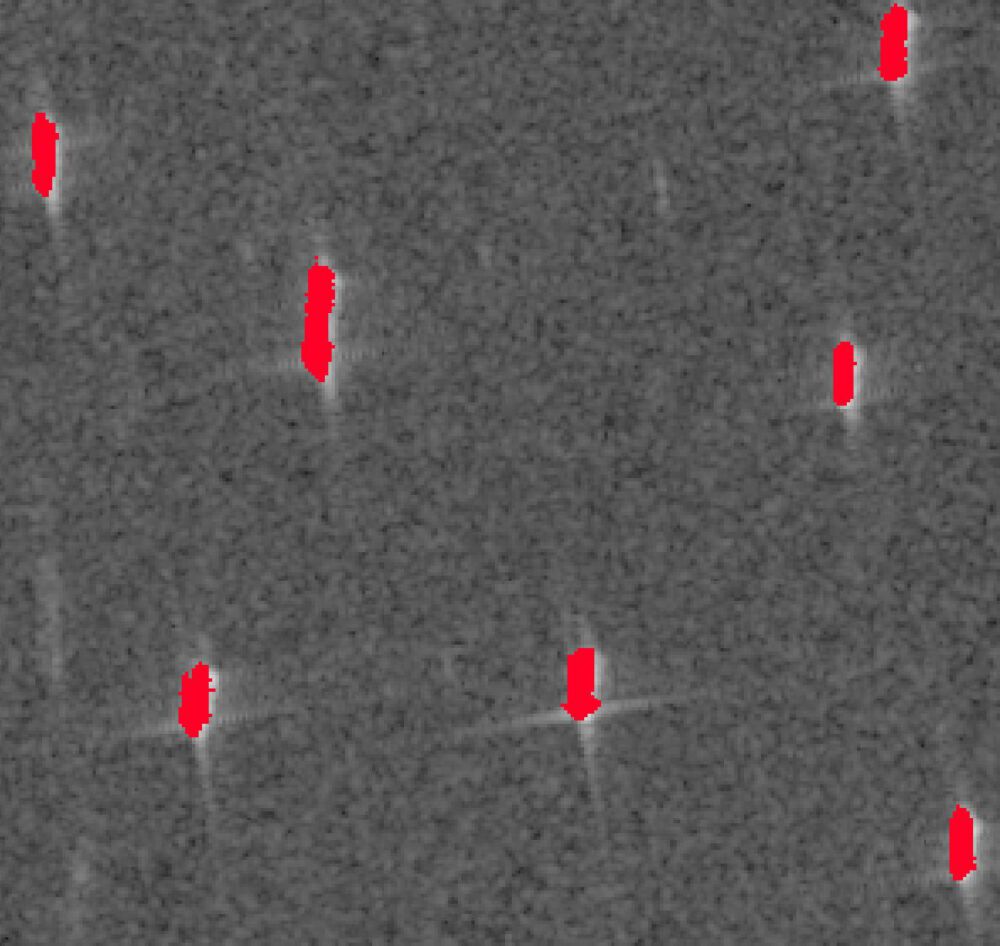
4.2 Final Result – Adapted Workflow
Looking purely at the results, not the method of the workflow itself, it seems that the workflow can be drastically shortened and made less complex while still obtaining good results.
Therefore, an adapted workflow was created, incorporating less steps and still yielding a good result.
The optimized workflow consists only of an erosion followed by an Otsu’s thresholding.
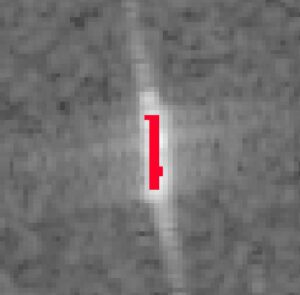
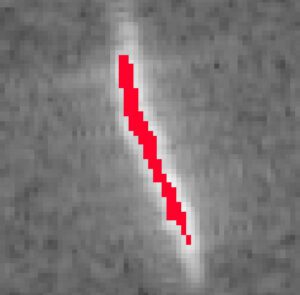
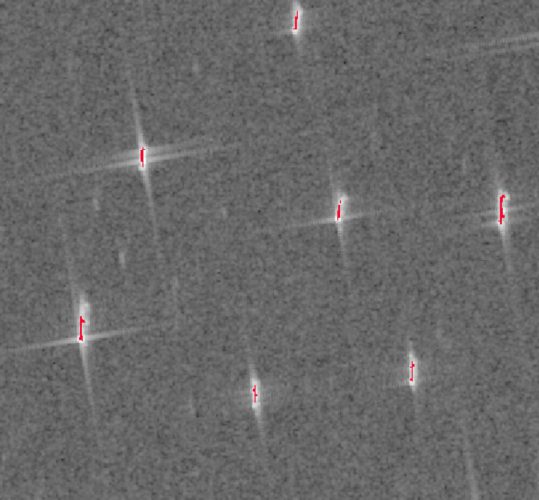
5. Conclusion
While at a first glance the methodology of the paper seemed quite straight-forward, upon further investigation it seemed more complex. Not only was it not possible for me to implement the correct thresholding algorithm (throwing off the results), but also some of the morphpological steps were hard to reproduce (mainly the multi-angular steps).
To me, it also seems that the workflow is overly complicated. While I can not verify my (or their) results due to missing ground-truth data, it seems like some steps could be streamlined while still obtaining good results.